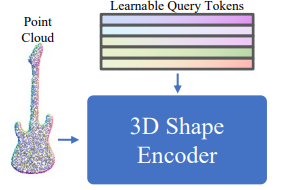
About
There is an interesting paper – CAD-Recode: Reverse Engineering CAD Code from Point Clouds -. I found it 2 days after when it is open 17 Dec 2024. https://arxiv.org/abs/2412.14042
This research is in the category of CAD data generation, similar to DeepCAD.
Unlike DeepCAD, it generates data using CADQuery and leverages synthetic data, achieving a highly rich three-dimensional representation.
There are several interesting aspects, and I’d like to introduce one of them.
( It seems that the strategy comes from Michelangelo in https://arxiv.org/abs/2306.17115)
3D Point Cloud Encoding in PointNet
PointNet’s encoding includes a process (T-Net) that estimates an appropriate transformation from the point cloud to enhance its spatial consistency. Additionally, it achieves order-invariant processing of point clouds by applying global pooling to the features. However, PointNet does not infer viewpoints; instead, it focuses on spatial transformations and order invariance.
Point Cloud Encoding in CAD-Recode
It takes a significantly different approach from PointNet’s encoding method.
- Apply uniform random sampling to downsample the input point clouds
- Apply lexicographic sorting by (z, y, x) coordinates
- Apply fourier positional encoding of coordinates and concatenating with point normals
- Employ a linear layer projection
Since it is using lexicographic sorting for each axis, the order is going to be neatly ordered.
And also, it is using positional encoding. I am not sure whether Fourier positional encoding was adopted specifically for using a Transformer, but, probably it is. The form of positional encoding in 3 dimensional space is like this.
Where f is frequency, and N is the order of encoding.
Their implementation is quite simple.
class FourierPointEncoder(nn.Module):
def __init__(self, hidden_size):
super().__init__()
frequencies = 2.0 ** torch.arange(8, dtype=torch.float32)
self.register_buffer('frequencies', frequencies, persistent=False)
self.projection = nn.Linear(54, hidden_size)
def forward(self, points):
x = points[..., :3]
x = (x.unsqueeze(-1) * self.frequencies).view(*x.shape[:-1], -1)
x = torch.cat((points[..., :3], x.sin(), x.cos()), dim=-1)
x = self.projection(torch.cat((x, points[..., 3:]), dim=-1))
return x
I will share the other components in the paper next time.